0. Abstract
Standard policy gradient method는 sample마다 한번의 gradient update를 하고 버린다.
PPO는
- minibatch를 갖고서 여러번의 update를 한다.
- TRPO의 일부 장점들을 가져온다.
- TRPO보다 더 간단하고 더 나은 sample-complexity를 갖는다.
1. Introduction
DQN은 discrete action space에만 적용이 된다.
Continuous action space의 경우 policy gradient method를 생각할 수 있다.
하지만 Vanilla policy rgadient method는 robustness와 data efficiency가 낮다.
Robustness는 hyperparameter tuning 없이 다른 문제들에 적용이 가능함을 말한다.
TRPO는 이 문제들을 해결했으나
- 너무 복잡하고
- architecture에 noise를 줄 수 없고 (dropout 불가능)
- value function과 policy간의 parameter 공유가 불가능하다.
PPO는 TRPO의 안정적 성능과 data efficiency를 챙기지만 일차미분만 활용하는 알고리즘이다.
Continuous control에선 다른 알고리즘을 압도하고 Atari에선 A2C보다 낫고 ACER와 비슷하다.
2. Background: Policy Optimization
2.1 Policy Gradient Methods
가장 흔히 사용되는 gradient estimator
은 시점에서 finite batch of samples에 대한 empirical average 은 시점에서 advantage function에 대한 estimator
- Sampling
- Optimizing value function
and policy function - repeat 1.
2번의 Opimization 과정에서 gradient descent를 여러번 하는 건 objective function이 수식적으로 정의가 안된 최적화이고 종종 collapse된다.
2.2 Trust Region Methods
이는 objective function에 linear approximation을 하고 constraint에 quadratic approximation을 한 후에 conjugate gradient algorithm을 사용해서 근사적으로 풀 수 있다.
TRPO의 논문에서 이론적으로 제시했던 바는
였지만, penalty term 대신 constraint를 했던 이유는
PPO는 추가적인 modification을 더해서 penalty term이 있는 꼴로 최적화할 것이다.
그 경우 일차미분으로 최적화가 가능해진다.
3. Clipped Surrogate Objective
미분을 했을 때 policy gradient가 나오는 대리 함수를 surrogate objective function이라 한다.
; 이 때 는 로 뽑은 것의 empirical average ; 이 때 는 로 뽑은 것의 empirical average
TRPO에서도 사용했던 두 번째 surrogate objective를 단순하게 gradient로 update하는 것은 확률의 ratio에 따라서 너무 큰 step을 갖게 된다.
어를 방지하기 위해,
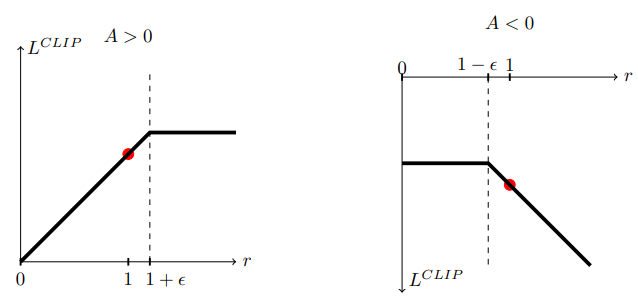
- objective를 향상시키는 policy의 변화에서
이 보다 큰 경우 gradient signal을 버리게 된다. - objective를 하향시키는 policy의 변화에서
이 보다 작은 경우 gradient signal을 버리게 된다.
더 직감적으로 말하면, advantage의 향상을 가져오는 sample 중에서 큰 policy의 변화가 있다면 update에 사용하지 않는다.
Note that
if if
4. Adaptive KL Penalty Coefficient
TRPO 논문에서 제안됐지만 penalty coefficient의 적절함이 없어서 못 쓴
를 휴리스틱하게 KL divergence가 크면
5. Algorithm
Asynchronous methods for deep reinforcement learning
은 (T-t) step TD error를 advantage function estimator로 쓴 것이다.
High-Dimensional Continuous Control Using Generalized Advantage Estimation
은 1~(T-t) step TD error의 exponential average를 advantage function estimator로 쓴 것이다.
Value function 학습엔 MSELoss를 사용한다.
추가로 sufficient exploration을 보장하기 위해 entropy bonus를 더하자.
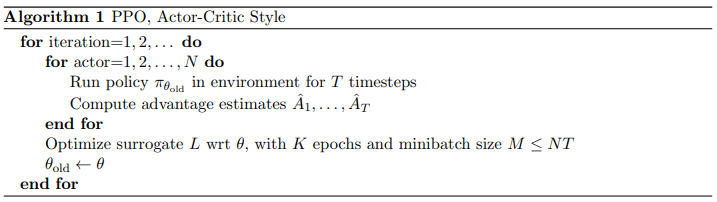